Unit 3. Training, Registering and Deploying Model¶
After designing and orchestrating the main pipeline, you can design and orchestrate the pipeline, train, register, and deploy the model in the sub-canvas of the ParallelFor operator to predict the wind power of each site.
Designing Pipelines¶
The processing logic for training, registering and deploying the models is given as follows:
Model training: Pass the site ID (the value of item, such as
abcde0001
) to the Python script for model training, and the model will be generated after the training is completed.Model creation: Use the item name to automatically create a model, and output the model name; if the model already exists, you don’t need to create it, and the model name will be outputted directly.
Model version staging: Stage the model version corresponding to the mode generated in Step 2.
Model testing: Before the model version is officially deployed, test the staged model version. The model version can be officially deployed only after it is qualified in the test.
Create a model deployment instance.
Model deployment: Deploy the model version that has qualified in the test online.
Double-click the ParallelFor operator and drag it to the sub-canvas of the ParallelFor operator, and the pipeline after orchestration is shown in the figure below:
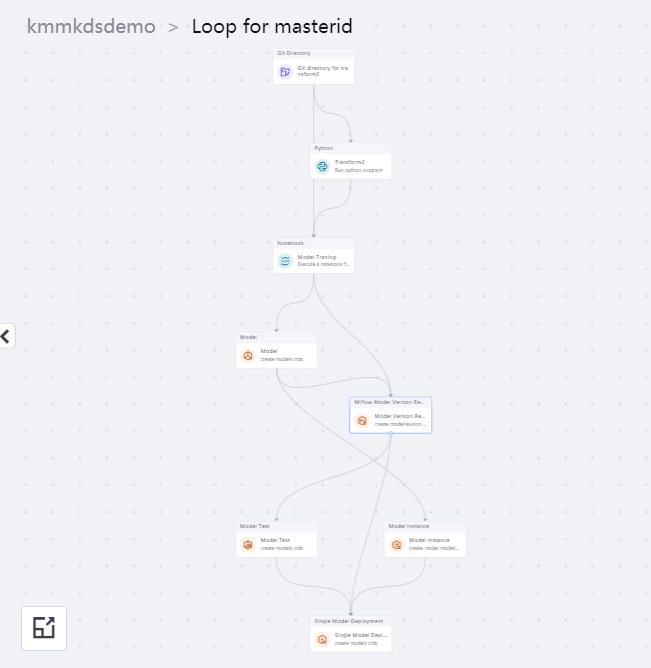
The configuration instructions for each operator orchestrated in the pipeline are given as follows:
Git Directory Operator¶
Name: Git directory for transform2
Description: pull the Python script for model training from the Git directory
Input parameters
Parameter Name |
Data type |
Operation Type |
Value |
---|---|---|---|
data_source_name |
String |
Declaration |
Name of the registered Git data source |
branch |
String |
Declaration |
master |
project |
String |
Declaration |
workspace1 |
paths |
List |
Declaration |
[“workspace1/kmmlds”] |
Output parameters
Parameter Name |
Value |
---|---|
workspace |
directory |
paths |
list |
An sample of operator configuration is given as follows:
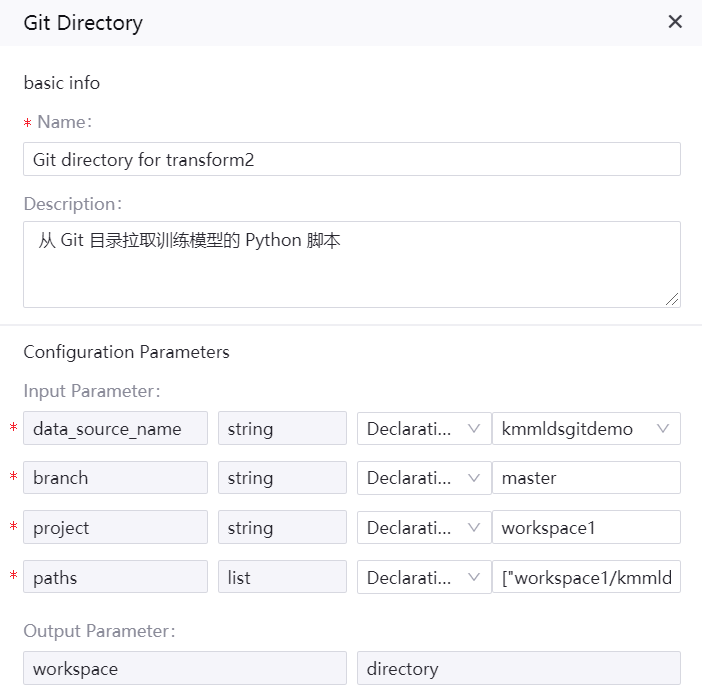
Python Operator¶
Name: Transform2
Description: format the input file and take it as the input of Notebook operator.
Input parameters
Parameter Name |
Data type |
Operation Type |
Value |
---|---|---|---|
workspace |
Directory |
Reference |
Git directory for transform2.workspace |
entrypoint |
String |
Declaration |
workspace1/kmmlds/transform2.py |
requirements_file_path |
String |
Declaration |
|
string_data |
variable |
Reference |
item |
Output parameters
Parameter Name |
Value |
---|---|
output_list |
list |
An sample of operator configuration is given as follows:

Notebook Operator¶
Name: Model Traning
Description: train the model
Input parameters
Parameter Name |
Data type |
Operation Type |
Value |
---|---|---|---|
workspace |
Directory |
Reference |
Git directory for transform2.workspace |
entrypoint |
String |
Declaration |
workspace1/kmmlds/train2.ipynb |
requirements_file_path |
String |
Declaration |
workspace1/kmmlds/requirements.txt |
env |
List |
Reference |
Transform2.output_list |
Output parameters
Parameter Name |
Value |
---|---|
mlflow_model_file_paths |
list |
An sample of operator configuration is given as follows:
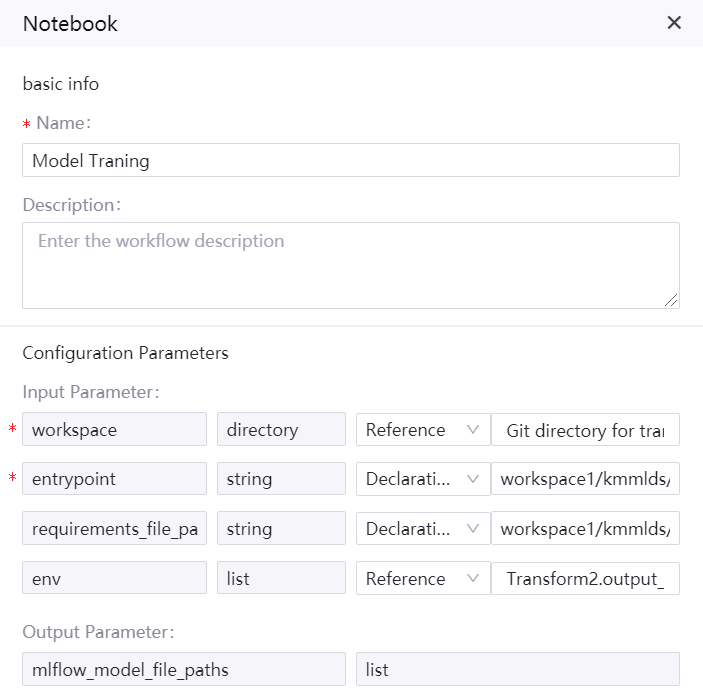
Model Operator¶
Name: Model
Description: register the model
Input parameters
Parameter Name |
Data type |
Operation Type |
Value |
---|---|---|---|
category |
String |
Declaration |
Predictor |
model_name |
String |
Reference |
item |
input_data_type |
String |
Declaration |
Text |
scope |
String |
Declaration |
Private |
technique |
String |
Declaration |
Regression |
usecase |
String |
Declaration |
Wind |
publisher |
String |
Declaration |
User_name (enter the username) |
input_format |
String |
Declaration |
Input parameters of the model feature in JSON format. See the sample. |
output_format |
String |
Declaration |
Model target output in JSON format, See the sample. |
interface |
String |
Declaration |
REST |
error_on_exist |
String |
Declaration |
false |
Output parameters
Parameter Name |
Data type |
---|---|
model_name_output |
string |
An sample of operator configuration is given as follows:
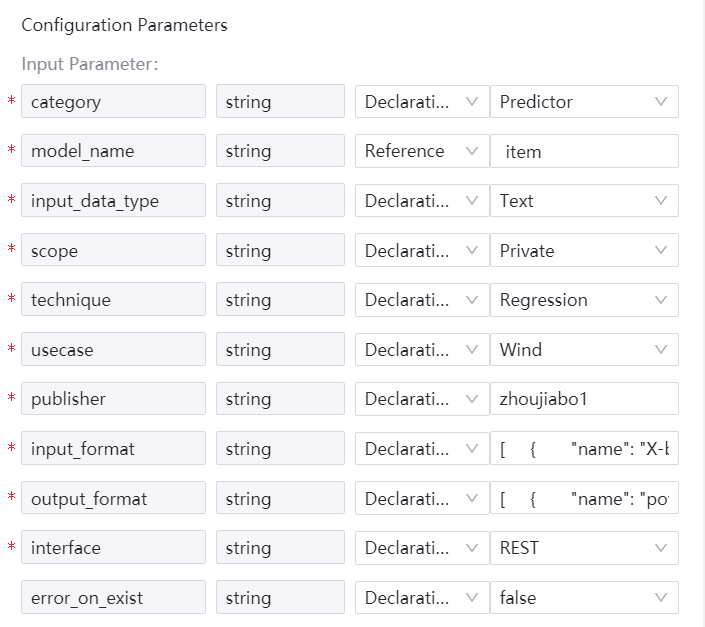
input_format sample
[{
"name": "X-basic.hour",
"dtype": "int",
"ftype": "continuous",
"range": [0, 23],
"annotations": "",
"repeat": null,
"defaultValue": 10
}, {
"name": "X-basic.horizon",
"dtype": "int",
"ftype": "continuous",
"range": [0, 49],
"annotations": "",
"repeat": null,
"defaultValue": 8
}, {
"name": "i-set",
"dtype": "int",
"ftype": "continuous",
"range": [0, 440],
"annotations": "",
"repeat": null,
"defaultValue": 300
}, {
"name": "EC-ws",
"dtype": "float",
"ftype": "continuous",
"range": [1, 2],
"annotations": "",
"repeat": null,
"defaultValue": "1.5"
}, {
"name": "EC-wd",
"dtype": "float",
"ftype": "continuous",
"range": [240, 300],
"annotations": "",
"repeat": null,
"defaultValue": 250
}, {
"name": "EC-tmp",
"dtype": "float",
"ftype": "continuous",
"range": [18, 30],
"annotations": "",
"repeat": null,
"defaultValue": 20
}, {
"name": "EC-pres",
"dtype": "float",
"ftype": "continuous",
"range": [820, 900],
"annotations": "",
"repeat": null,
"defaultValue": 850
}, {
"name": "EC-rho",
"dtype": "float",
"ftype": "continuous",
"range": [1, 2],
"annotations": "",
"repeat": null,
"defaultValue": 1
}, {
"name": "EC-dist",
"dtype": "float",
"ftype": "continuous",
"range": [12, 100],
"annotations": "",
"repeat": null,
"defaultValue": 14
}, {
"name": "GFS-ws",
"dtype": "float",
"ftype": "continuous",
"range": [1, 2],
"annotations": "",
"repeat": null,
"defaultValue": 1
}, {
"name": "GFS-wd",
"dtype": "float",
"ftype": "continuous",
"range": [40, 300],
"annotations": "",
"repeat": null,
"defaultValue": 50
}, {
"name": "GFS-tmp",
"dtype": "float",
"ftype": "continuous",
"range": [18, 20],
"annotations": "",
"repeat": null,
"defaultValue": 19
}, {
"name": "GFS-pres",
"dtype": "float",
"ftype": "continuous",
"range": [840, 900],
"annotations": "",
"repeat": null,
"defaultValue": 850
}, {
"name": "GFS-rho",
"dtype": "float",
"ftype": "continuous",
"range": [1, 2],
"annotations": "",
"repeat": null,
"defaultValue": 1
}, {
"name": "GFS-dist",
"dtype": "int",
"ftype": "continuous",
"range": [12, 100],
"annotations": "",
"repeat": null,
"defaultValue": 20
}, {
"name": "sequence",
"dtype": "int",
"ftype": "continuous",
"range": [1, 26901],
"annotations": "",
"repeat": null,
"defaultValue": 20
}]
output_format sample
[{
"name": "power",
"dtype": "float",
"ftype": "continuous",
"range": [],
"annotations": "",
"repeat": null,
"defaultValue": 0
}]
Mlflow Model Version Register Operator¶
Name: Model Version Register
Description: stage the model version
Input parameters
Parameter Name |
Data type |
Operation Type |
Value |
---|---|---|---|
input_data |
String |
Declaration |
Model version parameter input. See the sample. |
version_rule |
String |
Declaration |
time |
annotation |
String |
Declaration |
test |
architecture |
String |
Declaration |
x86 |
coprocessor |
String |
Declaration |
None |
env_param |
List |
Declaration |
[] |
framework |
String |
Declaration |
sklearn |
language |
String |
Declaration |
python3 |
model_reference |
String |
Reference |
Model.model_name_output |
publisher |
String |
Declaration |
User_name (name of the model version creator) |
minio_paths |
List |
Reference |
Model Traning.mlflow_model_file_paths |
Output parameters
Parameter Name |
Parameter type |
---|---|
create_model_revision |
String |
model_revision_name |
String |
model_builder_name |
String |
An sample of operator configuration is given as follows:
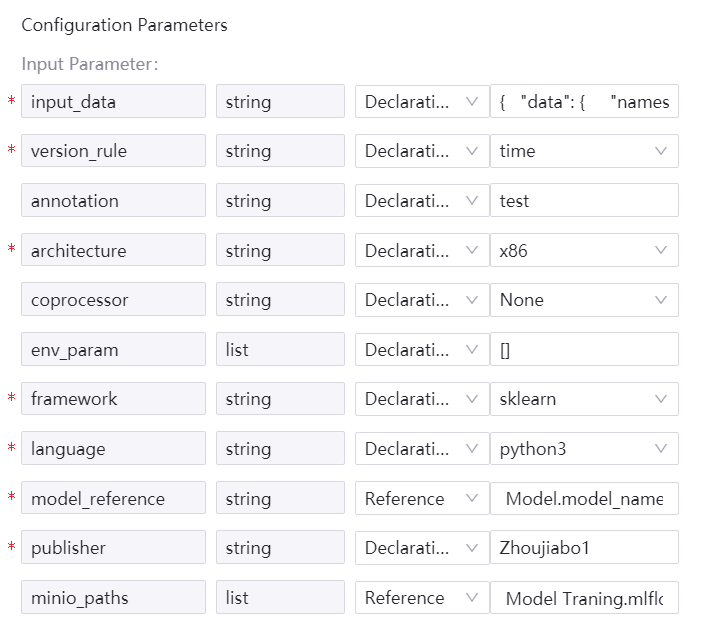
Input_data sample
{
"data": {
"names": ["sequence", "X-basic.hour", "X-basic.horizon", "i-set", "EC-ws", "EC-wd", "EC-tmp", "EC-pres", "EC-rho", "EC-dist", "GFS-ws", "GFS-wd", "GFS-tmp", "GFS-pres", "GFS-rho", "GFS-dist"],
"ndarray": [
[20000, 11, 37, 1, 2, 257, 18, 85, 0, 15, 1, 6, 20, 879, 1, 59],
[200500, 1, 3, 1, 2, 57, 18, 85, 0, 15, 1, 1, 20, 879, 1, 59]
]
}
}
Model Test Operator¶
Name: Model Test
Description: test the model version
Input parameters
Parameter Name |
Data type |
Operation Type |
Value |
---|---|---|---|
input_data |
String |
Declaration |
Enter the model testing data in JSON format. See the sample. |
model_builder |
String |
Reference |
Model Version Register.model_builder_name |
Output parameters
Parameter Name |
Parameter type |
---|---|
create_model_test |
String |
model_test_output |
String |
An sample of operator configuration is given as follows:
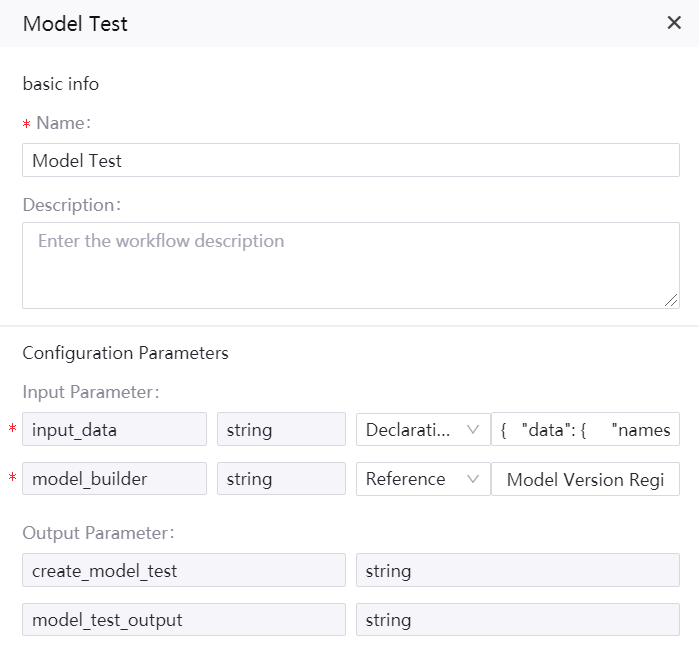
Input_data sample
{
"data": {
"names": ["sequence", "X-basic.hour", "X-basic.horizon", "i-set", "EC-ws", "EC-wd", "EC-tmp", "EC-pres", "EC-rho", "EC-dist", "GFS-ws", "GFS-wd", "GFS-tmp", "GFS-pres", "GFS-rho", "GFS-dist"],
"ndarray": [
[20000, 11, 37, 1, 2, 257, 18, 85, 0, 15, 1, 6, 20, 879, 1, 59],
[200500, 1, 3, 1, 2, 57, 18, 85, 0, 15, 1, 1, 20, 879, 1, 59]
]
}
}
Single Instance Operator¶
Name: Model Instance
Description: model deployment instance
Input parameters
Parameter Name |
Data type |
Operation Type |
Value |
---|---|---|---|
name |
String |
Declaration |
Enter the name of the model deployment instance (e.g. abctest) |
resource_pool |
String |
Declaration |
Select the deployment model resource pool |
model_name |
String |
Reference |
Model.model_name_output |
labels |
List |
Declaration |
(Optional) enter the tag of the model deployment instance |
description |
String |
Declaration |
(Optional) enter the description of the model deployment instance |
deploy_mode |
String |
Declaration |
ONLINE |
error_on_exist |
String |
Declaration |
false |
Output parameters
Parameter Name |
Parameter type |
---|---|
instance_name_output |
String |
An sample of operator configuration is given as follows:
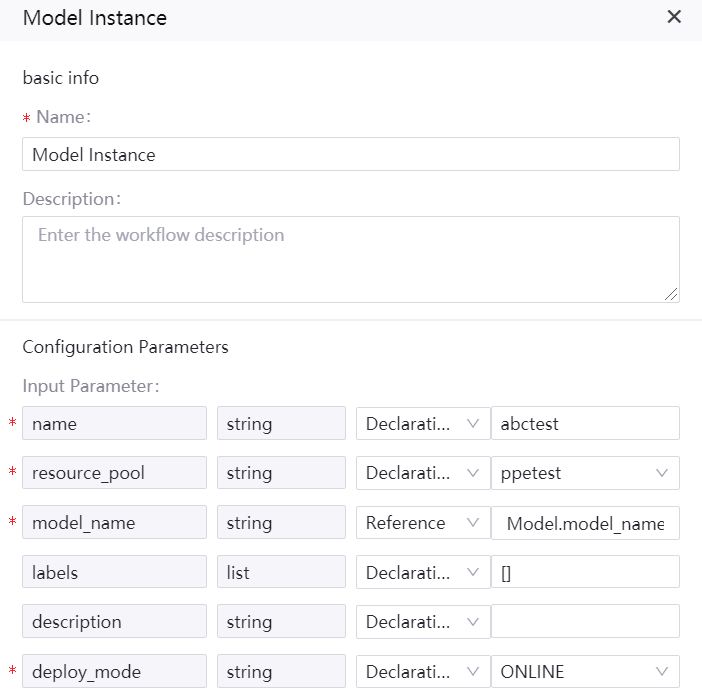
Single Model Deployment Operator¶
Name: Single Model Deployment
Description: model version deployment
Input parameters
Parameter Name |
Data type |
Operation Type |
Value |
---|---|---|---|
model_revision |
String |
Reference |
Model Version Register.model_revision_name |
instance_name |
String |
Declaration |
Model Instance.instance_name_output |
request_cpu |
Number |
Declaration |
0.5 |
request_memory |
Number |
Declaration |
0.5 |
limit_cpu |
Number |
Declaration |
1.0 |
limit_memory |
Number |
Declaration |
1.0 |
timeout |
Number |
Declaration |
360 |
Output parameters
Parameter Name |
Parameter type |
---|---|
create_model_deployment |
String |
An sample of operator configuration is given as follows:
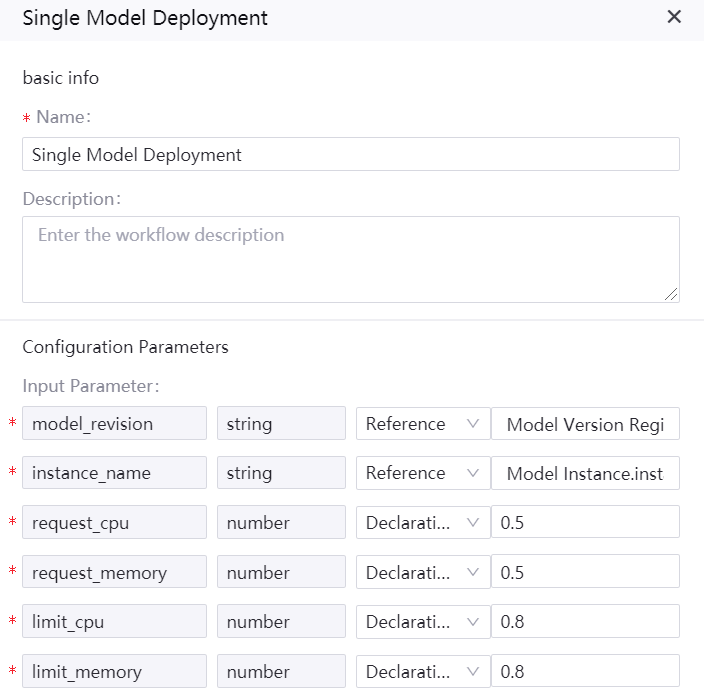